Overview
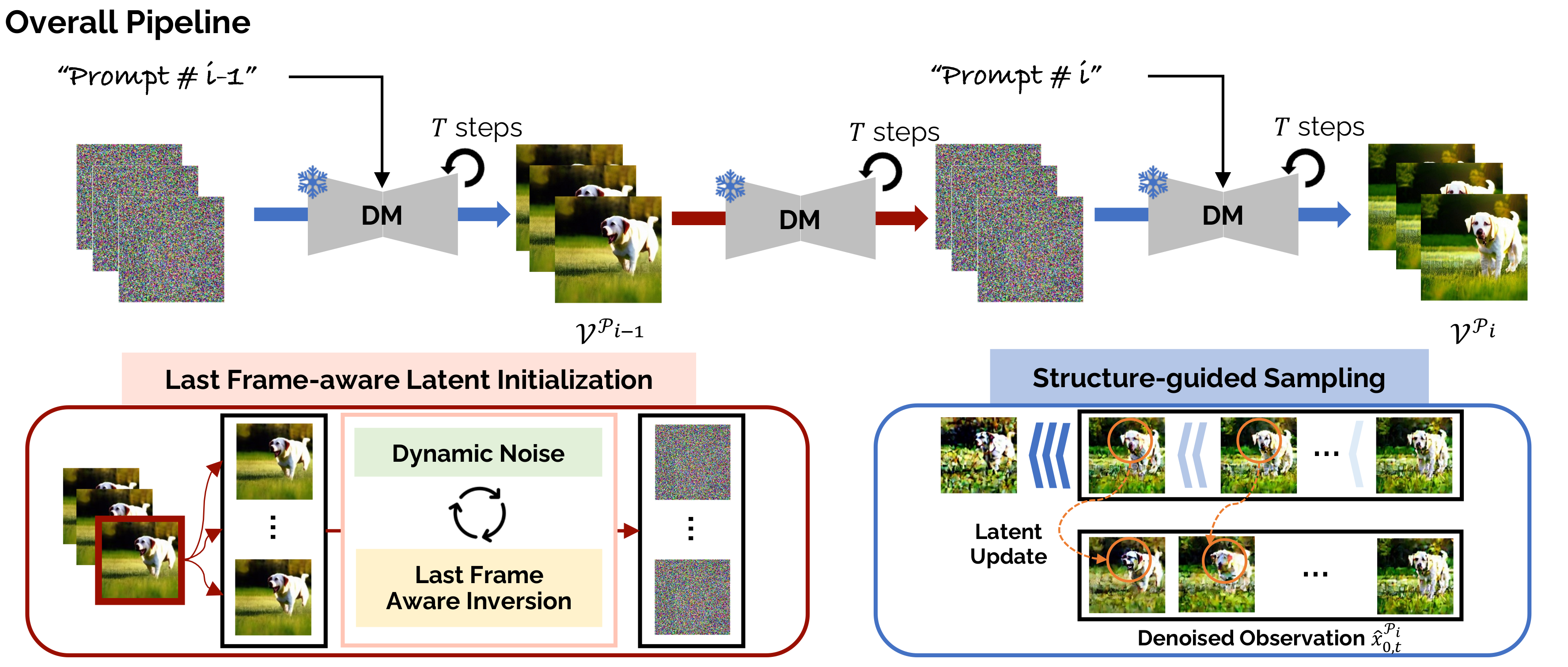
MTVG synthesizes the consecutive video clips corresponding to distinct prompts. The overall pipeline comprises two major components: last frame-aware latent initialization and structure-guided sampling. First, in the last frame-aware latent initialization, the pre-trained text-to-video generation model adopts the repeated frame as an input to invert into the initial latent code with two novel techniques: dynamic noise and last frame-aware inversion. Second, structure-guided sampling enforces continuity within a video clip by updating the latent code.